Michelle Espinosa is Senior Director of AI/ML Testing Services, Applause.
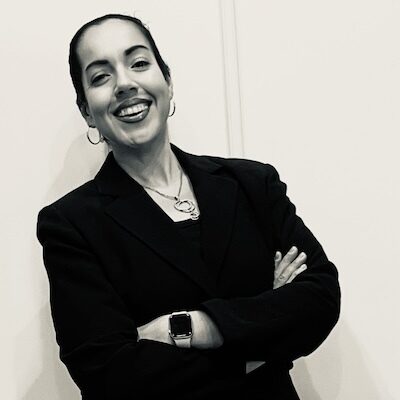
Data detective by day, doctoral researcher by night – Michelle spent 13 years wearing multiple hats in the nonprofit sector, where she focused on recidivism reduction, and the last 9 years wearing multiple hats in the tech industry. In her role as Senior Director at Applause, Michelle leads the data analytics and AI/ML Insights practice. Michelle is an expert on topics across economics, business administration, data science, AI, complexity science, and cybersecurity.
The ability of Generative AI (Gen AI) applications such as ChatGPT to perform a variety of tasks at a much faster rate than people represents a significant opportunity for businesses.
However, such tools can pose significant risks if the data sets they’re built on are producing very biased, incorrect, or even harmful outcomes.
Bias in Gen AI is a very real problem. A recent survey revealed that 90 percent of respondents have some level of concern around the effect of bias on the accuracy, relevance, or tone of AI-generated content. Of these, nearly half (47%) said they experienced content or responses they considered to be biased.
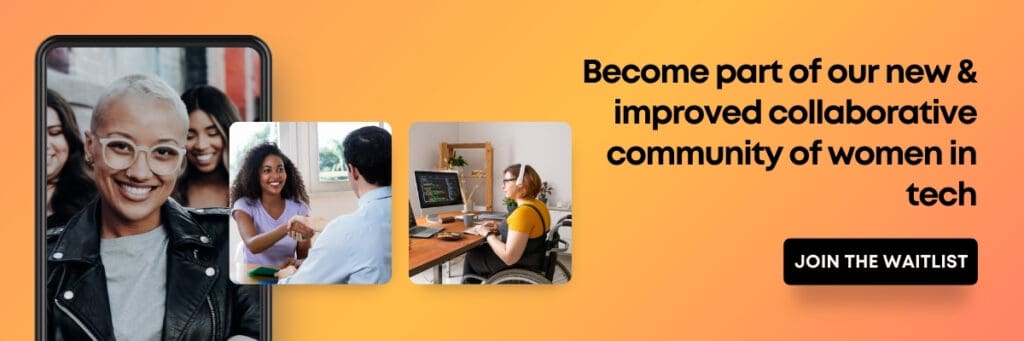
The problem of misrepresentation
When you leave certain voices out of AI development and testing, you end up with products that work well for some groups but not for others. Using a Pokémon metaphor: If everyone designing your products is a Charizard, you’ll have great products for fire-breathing dragons, but they may be less usable for Pikachu. A Deep Dive into Language Learning Models ‘LLM’ can help ensure diverse perspectives are included in product design.
A commonly cited example is the ‘gender design gap’. A 2023 World Economic Forum report found that women made up only around a quarter (26%) of workers in data and AI roles globally. Ignoring a female perspective can result in products that work better for men. Yvonne Lin, writing in Fast Company, explains the phenomenon, saying: “the easiest person to understand and design for is yourself. And when ‘yourself’ is 85% male, that’s whom you tend to design for the best.”
What minimising bias actually means… in theory
Because such tools reflect the biases of their creators, it is impossible to eliminate bias from AI regardless of how carefully the training data is curated. Therefore, the goal should be to minimise harmful outcomes rather than eliminate them entirely. When talking about bias, it is important to note that the stratification of race, gender, culture, age, ability, cognition, language, dialect and creed differ by community. Debates around bias in AI typically focus on the stratification used in the United States and Europe.
Training data is, by virtue of its existence, historical information. Since history is told by people with the most dominant voices in society, machine learning models reflect the systemic biases that existed at the point when the data is sampled. The lack of representation of marginalised voices in training data is one reason why efforts to remove bias have seen limited success. The mere fact that I type this article without fear of harm or retribution implies that I am already in a privileged position in society and need not fear being harmed by a chatbot’s response.
So, if the goal is to minimise harmful outcomes caused by bias, rather than to eliminate them entirely, then how do we measure ‘minimum’ and how do we measure ‘harm’? One might argue that minimizing harm translates to harming the fewest people possible. It’s a very utilitarian perspective. However, developers that follow this approach ultimately end up perpetuating the bias they set out to remove. This is because, by prioritising helping the most people, you ultimately end up de-prioritising helping groups that are already marginalised.
… And what it means in practice
There is no cheat sheet for minimising bias. It requires bringing about real systematic change, both in society and organisations developing AI. But that doesn’t mean companies can’t start somewhere. Here are some suggestions:
- Rethink hiring practices
Companies struggling to attain or retain diversity in their organisations should start with examining their hiring practices. Look for voices that are missing in the room. People who hire for cultural fit, or those who fit the hiring manager’s idea of the ideal job candidate, are likely to hire those most like themselves. Hiring managers should seek to round out a team’s skill base, backgrounds, perspectives, experiences, personality traits, interests, etc. This intentional approach will result in a balanced workforce where each employee possesses a unique strength that contributes to overall organisational growth and success.
- Beware the queen-bee syndrome
Self-group distancing is a phenomenon that occurs when members of a stigmatised or marginalised group distance themselves from their own group in an attempt to be seen as more like the dominant group. The behavior stems from social identity threat, where individuals suspect that they are valued less because of their social identity. Self-group distancing manifests in different ways. It sometimes leads to disparately harsh treatment of other members of the same group in order to preserve individual mobility, or to avoid appearing to give favoritism to other group members. “Queen bee syndrome” is a colloquial term for self-group distancing among women.
If we think of our careers and lives as a collective mountain climb, then once you reach the mountain top, who do you reach back and help? Do you offer a hand to the person with the easiest climb or the person struggling the most?
- Include diverse perspectives in testing
To minimise the risk of bias, companies must ensure diverse human perspectives are represented both in AI training data, but also in testing efforts. Through crowdtesting, companies can ensure their Gen AI applications are tested by people from diverse communities all over the world — and it’s these people who can ultimately say whether products work for them or not.